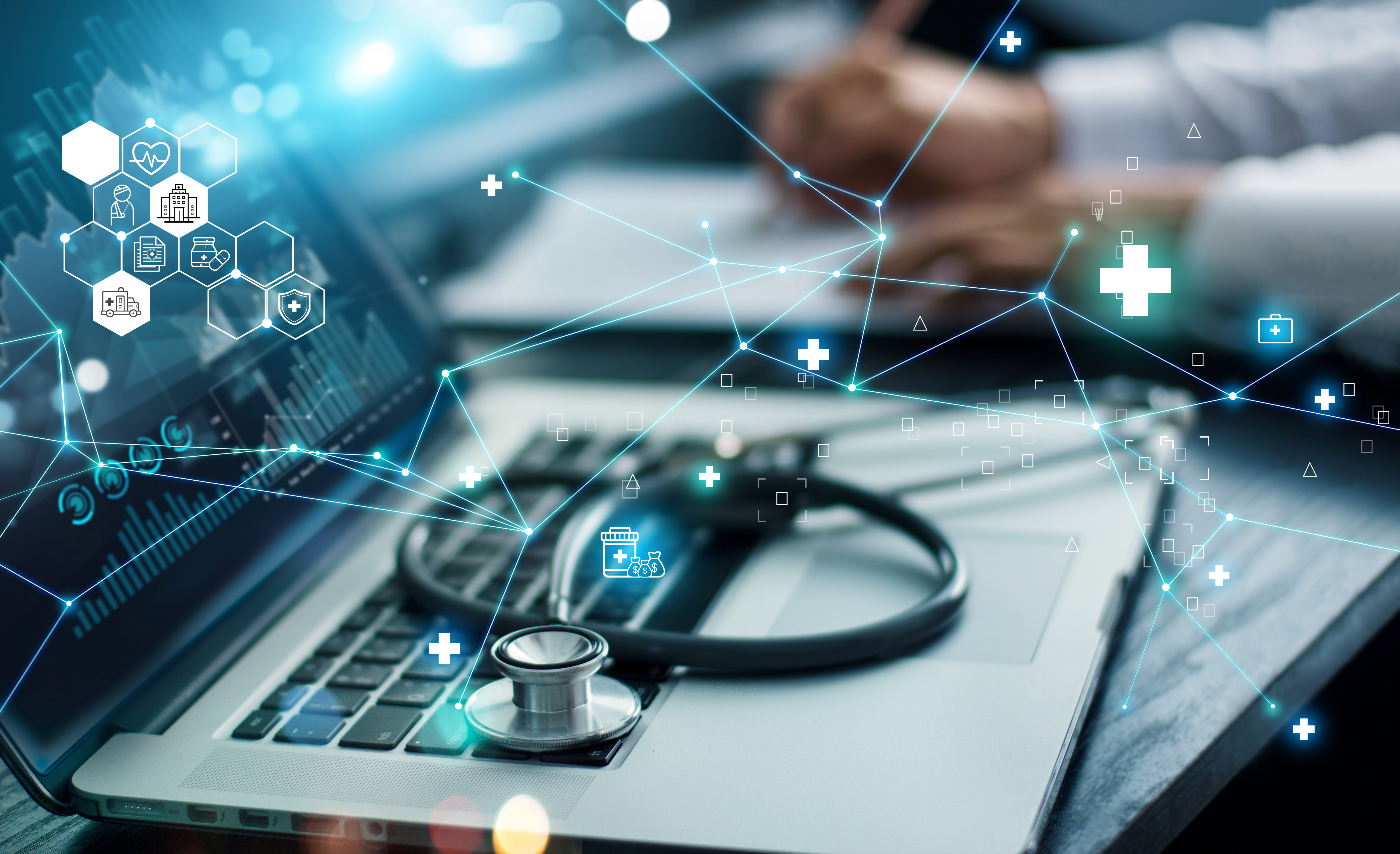
A guide for healthcare leaders on ways to leverage AI in mental health care
Heather Grigo, MD
May 2025
The U.S. is currently experiencing a mental health crisis with rising levels of unmet behavioral health needs across all ages. It is estimated that more than one in five U.S. adults (23.1%) live with a mental illness1. Additionally, 17.3% of people aged 12 and older had a substance use disorder in the past year2. Depression is on the rise, with recent data indicating that its prevalence among U.S. adolescents and adults has increased by 60% over the past decade3. Among adolescents aged 12 to 17, 13.4% had serious thoughts of suicide, 6.5% made a suicidal plan, and 3.7% attempted suicide in the past year4. Spending has also trended upward: from 2019 to 2022 utilization and spending rates for mental health care services among commercially insured adults increased by 38.8% and 53.7%, respectively5. The ability to effectively address this crisis may partly depend on the development and deployment of innovative technologies capable of meeting these complex and widespread challenges.
Artificial intelligence (AI) has the potential to transform mental health care by providing insights and solutions that were previously unattainable through conventional methods. The integration of AI into mental health care dates back to the mid-20th century, when scientists envisioned using robots to imitate cognitive processes. This was followed by the development in the 1960s of a rule-based chatbot (ELIZA) that simulated a Rogerian psychotherapist, the creation of rule-based AI systems designed to mimic human expertise in the 1980s, and then in the late 20th century with computerized cognitive-behavioral therapy programs6. The evolution of AI’s role in mental health care has increased exponentially since the early 21st century. We have seen advances in the early identification of mental health conditions and development of treatment recommendations, as well as the use of specific tools to conduct virtual therapy6. By integrating artificial intelligence into mental health care, there is an opportunity to address some of the most pressing challenges in the field. Whether improving access to care with AI-driven therapy chatbots, reducing clinicians’ administrative burden by incorporating ambient AI listening tools into their practice, or enhancing the ability to diagnose patients using AI-enabled Clinical Decision Support Systems, the application of AI has the potential to profoundly impact patients, clinicians, and the mental health care system as a whole.
Improving Access to Care with AI-Driven Chatbots
The significant increase in demand for mental health services is placing a strain on already taxed resources. In addition, there is a nationwide shortage of providers. According to the National Center for Health Workforce Analysis, as of December 2023, over half of the U.S. population lives in a Mental Health Professional Shortage Area7. There is also a critical workforce shortage of child and adolescent psychiatrists. Data from the American Academy of Child and Adolescent Psychiatry8 shows that on average, there are only 14 child and adolescent psychiatrists for every 100,000 children in the U.S., with most states in severe shortage. The resulting extended wait times for appointments or long-distance travel for medically necessary care delays care and increases the burden on parents. Prompt access to mental health care is critical, particularly when demand is already high. Broadening the availability of mental health care to those without access – or who prefer alternative options due to perceived stigma or other reasons – could reduce symptom severity and improve functioning, thereby mitigating the risk of crises and hospitalizations.
One way to increase access to services is through the use of AI-driven therapy chatbots. These interventions use natural language processing (NLP) to interpret the meaning and intent behind the user’s input. By classifying real-time, multimodal input according to the user’s emotional state, they can simulate human conversation and respond empathically, offering opportunities for therapeutic dialogue9. Due to their convenience, they also enable the delivery of personalized mental health interventions at scale. This scalability is crucial in addressing the current shortage of mental health professionals.
Cognitive-behavioral therapy (CBT)-based digital therapeutics and rule-based AI chatbots have already shown effectiveness in treating depression and anxiety10. One study found a chatbot was able to establish a working alliance comparable to traditional, human-delivered services across different treatment modalities11. AI-driven chatbots have also been developed to provide therapy for children with autism spectrum disorder12. These tools can teach emotional recognition and social skills by analyzing a child’s facial expressions and adjusting their interactions accordingly.
Recently, the first randomized controlled trial demonstrating the effectiveness of a fully generative-AI-powered therapy chatbot for treating clinical-level mental health symptoms showed that users experienced greater reductions in depression, anxiety and clinically high-risk feeding and eating disorder (CHR-FED) symptoms compared to the waitlist control group13. In addition, during the four-week trial, users demonstrated sustained engagement and reported that their alliance with the chatbot was comparable to that of human therapists. Notably, patients also appeared to have an overall positive perception of using chatbots for mental health conditions14. Since perception can influence the adoption of a technology, high levels of perceived usefulness highlights the ongoing potential impact of chatbots in mental health care.
Reducing Administrative Burden with Ambient AI Listening Tools
The complexity and time-consuming nature of administrative tasks produces a significant burden on providers, many of whom already have schedules at capacity. According to the American Psychiatric Association, most psychiatrists spend just 60% of their time with patients. As providers struggle to balance time spent seeing patients with administrative responsibilities-such as documenting patient care and completing prior authorization requests, this can lead to decreased job satisfaction and even burnout. A recent survey of practicing physicians by the American Medical Association found that physicians and their staff spend an average of 13 hours each week completing prior authorizations, and 89% of physicians surveyed reported that these requirements somewhat or significantly increase physician burnout15. At a time of already limited provider availability, physician burnout could further exacerbate existing workforce shortages.
Automatic note generation using HIPAA-compliant ambient AI listening tools has the ability to record clinical interactions and is increasingly integrated directly into providers’ electronic health record systems. Adopting these tools enables mental health clinicians to improve efficiency by reducing documentation time, allowing them to focus on what matters most: personalized care. In addition, by saving time on administrative activities, providers may be able to see more patients, thereby improving access to mental health services through increased clinical productivity. According to a qualitative study evaluating physician perspectives on an ambient AI scribe pilot, those interviewed had predominantly positive views on the impact of ambient AI scribes on temporal demand, work-life integration, patient engagement, and overall workload. However, there were predominantly negative perspectives on note construction, specifically accuracy and style16.
There are also applications for AI in automating other administrative tasks such as billing, scheduling, and basic patient communication17. When used responsibly and ethically, AI-enabled tools can optimize the prior authorization process, allowing providers to spend less time on insurance-related tasks. By streamlining many of the administrative burdens that weigh down the healthcare system, AI integration has the potential to reduce burnout in the existing mental health workforce—thereby addressing one of the major challenges currently facing the provision of mental health care in the United States.
Enhancing Clinical Decision-Making Using AI-Enabled Systems
The ability of artificial intelligence to aid in detecting specific mental health conditions has the potential to improve diagnostic accuracy. Certain AI-enabled Clinical Decision Support Systems (AI-CDSS) can assist mental health practitioners in diagnosing or predicting the onset of conditions18. The Cognoa ASD Diagnosis Aid and EarliPoint System are FDA Class II devices designed for use by health care providers to assist in the diagnosis of autism spectrum disorder (ASD) in children. The Cognoa ASD Diagnosis Aid uses a machine learning algorithm to predict autism spectrum disorder based on clinical data and videos uploaded by caregivers through a mobile application. AI is used to analyze the combined data and produce a value that, when compared to pre-defined thresholds, determines if a diagnosis of ASD is present. The EarliPoint System assists healthcare providers in diagnosing ASD in children between 16 and 30 months of age who are at risk for developmental delays by tracking the child’s visual reactions to social information presented in videos.
One AI-CDSS device, Limbic Access, is currently integrated within the National Health Service in the United Kingdom. It uses conversational data collected through a mobile application. A thorough symptom profile is created for each user, and the generated insights regarding the most likely diagnoses is presented to the treating clinician as a digital report to aid in diagnostic and treatment decision-making19. Researchers have also developed a combination of machine learning and deep learning algorithms to detect schizophrenia, depression and anxiety, bipolar disorder, posttraumatic stress disorder, anorexia nervosa, and attention-deficit/hyperactivity disorder20. Although results can vary in terms of predictive accuracy and precision, all studies that implemented deep learning methods reported an accuracy of at least 63.62%. By improving diagnostic accuracy within mental health care, clinicians may be able to make more informed and timely decisions, which could ultimately improve patient outcomes by mitigating the progression of mental health conditions.
In terms of therapeutic outcomes, several studies have evaluated the ability of machine learning models to develop personalized care recommendations based on predicted clinical response to treatment, with promising results across a range of psychiatric conditions21,22. A recent meta-analysis of existing literature evaluated 14 studies investigating the impact of machine learning models, deep learning models, and hybrid models combining multiple AI approaches on both diagnostic accuracy and therapeutic efficacy23. The results showed that AI models have a robust impact on both diagnostic accuracy and therapeutic outcomes (with pooled effect sizes of 0.85 for diagnostic accuracy and 0.84 for therapeutic efficacy). Among the AI methodologies reviewed, machine learning models demonstrated the highest diagnostic and therapeutic performance.
Ethical Implications and Challenges
Despite many promising applications, the integration of AI in mental health care presents clear challenges. There are ethical considerations surrounding data privacy as more clinicians incorporate AI tools into clinical care. It is an imperative to protect the privacy, security and consent of patients and health care professionals, particularly given the rapid advancement and widespread adoption of AI, especially as regulations struggle to keep pace.
Some studies have also identified risks associated with conversational agent interventions (CAIs), including chatbots. Misunderstandings on the part of the CAI could result in ineffective or even harmful interventions, and there may be no built-in crisis warning system to respond in the event of an emergency24. Additionally, there is a growing need for transparency in the development of AI technologies. Machine learning systems in health care may be subject to algorithmic bias25. A balanced approach is necessary to ensure the safety, quality, and fairness of AI systems. This includes the refinement of AI models for broader and more diverse populations. The impact of AI on healthcare costs, access, and outcomes also warrants further research and evaluation to guide future development. Finally, in order to ensure responsible use, AI should serve as a method to augment and enhance, not replace, sound clinical decision-making and judgement.
The American Psychiatric Association recognizes the potentially revolutionary role of AI in automating elements of medicine to improve both clinician and patient experience and generate better outcomes. However, it urges caution in the application of untested technologies within clinical medicine26. Multiple efforts are underway to pass international laws and create guidelines for the responsible use of AI in healthcare, including initiatives by the World Health Organization27. With the engagement of key stakeholders, the National Academy of Medicine is currently working to develop an AI Code of Conduct framework. Their goal is the intentional design of the future of AI-enabled health, health care and biomedical science that advances the vision of health and well-being for all28.
Conclusion and Future Outlook
The mental health crisis in the United States continues to intensify with growing prevalence of mental health conditions, increasing demand for care, and a shortage of clinical providers. Artificial intelligence offers a powerful set of tools to reimagine how care is delivered, accessed, and optimized. We are already seeing the promise of AI-enabled technologies for increasing therapy access, improving clinical efficiency, and enhancing diagnostic and treatment capabilities.
While the benefits are compelling, this transformative potential should be balanced with a clear understanding of the ethical and practical risks. Healthcare leaders need to consider issues such as data privacy, algorithmic bias, system transparency, and the absence of crisis safeguards. Above all, AI must remain a complement to, not a replacement for, human clinical judgment in all areas that involve medical decision-making.
To fully realize AI’s potential in mental health care, ongoing interdisciplinary collaboration is essential. This includes not only clinicians, patients, and AI developers, but also policymakers, ethicists, professional societies, and the broader public, who can advocate for and shape robust regulatory frameworks and standards. Continued research and impact assessments will also play a crucial role in refining these technologies for real-world use.
Artificial intelligence represents a promising frontier in the evolution of mental health care. If deployed strategically and ethically, it may help bridge longstanding gaps in access to care, improve clinical outcomes, and strengthen the resilience of the mental health system for the future.
References:
- National Institutes of Mental Health: 2022 National Survey on Drug Use and Health, https://www.nimh.nih.gov/health/statistics/mental-illness
- National Institutes of Mental Health: 2022 National Survey on Drug Use and Health, https://www.nimh.nih.gov/health/statistics/mental-illness
- National Center for Health Statistics: Depression Prevalence in Adolescents and Adults: United States, August 2021-August 2023, ttps://www.cdc.gov/nchs/products/databriefs/db527.html
- Cantor JH, McBain RK, Ho P, Bravata DM, Whaley C. Telehealth and In-Person Mental Health Service Utilization and Spending, 2019 to 2022. JAMA Health Forum. 2023;4(8):e232645, doi:10.1001/jamahealthforum.2023.2645
- National Institutes of Mental Health: 2022 National Survey on Drug Use and Health, https://www.nimh.nih.gov/health/statistics/mental-illness
- Cantor JH, McBain RK, Ho P, Bravata DM, Whaley C. Telehealth and In-Person Mental Health Service Utilization and Spending, 2019 to 2022. JAMA Health Forum. 2023;4(8):e232645, doi:10.1001/jamahealthforum.2023.2645
- 6. Olawade DB, Wada OZ, Odetayo A, David-Olawade AC, Asaolu F, Eberhardt J. Enhancing mental health with Artificial Intelligence: Current trends and future prospects. Journal of Medicine, Surgery, and Public Health. 2024; Volume 3, Article 100099, https://doi.org/10.1016/j.glmedi.2024.100099
- 7. National Center for Health Workface Analysis: HRSA Behavioral Health Workforce, 2023, htps://bhw.hrsa.gov/sites/default/files/bureau-health-workforce/Behavioral-Health-Workforce-Brief-2023.pdf
- 8. American Academy of Child & Adolescent Psychiatry: Severe Shortage of Child and Adolescent Psychiatrists Illustrated in AACAP Workforce Maps, 2022, https://www.aacap.org/AACAP/zLatest_News/Severe_Shortage_Child_Adolescent_Psychiatrists_Illustrated_AACAP_Workforce_Maps.aspx
- 9. He Y, Yang L, Qian C, Li T, Su Z, Zhang Q, Hou X. Conversational Agent Interventions for Mental Health Problems: Systematic Review and Meta-analysis of Randomized Controlled Trials. J Med Internet Res. 2023;25:e43862, doi: 10.2196/43862
- 10. Fitzpatrick KK, Darcy A, Vierhile Delivering Cognitive Behavior Therapy to Young Adults With Symptoms of Depression and Anxiety Using a Fully Automated Conversational Agent (Woebot): A Randomized Controlled Trial. JMIR Ment Health. 2017;4(2):e19, doi: 10.2196/mental.7785
- 11. Darcy A, Daniels J, Salinger D, Wicks P, Robinson A. Evidence of Human-Level Bonds Established With a Digital Conversational Agent: Cross-sectional, Retrospective Observational Study. JMIR Form Res. 2021 May 11;5(5):e doi: 10.2196/27868.
- 12. Olawade DB, Wada OZ, Odetayo A, David-Olawade AC, Asaolu F, Eberhardt J. Enhancing mental health with Artificial Intelligence: Current trends and future prospects. Journal of Medicine, Surgery, and Public Health. 2024; Volume 3, Article 100099, https://doi.org/10.1016/j.glmedi.2024.100099
- 23. Heinz MV, Mackin DM, Trudeau BM, Bhattacharya S, Wang Y, Banta HA, Jewett AD, Salzhauer AJ, Griffin TZ , Jacobson NC. Randomized Trial of a Generative AI Chatbot for Mental Health Treatment. NEJM AI 2025;2(4), doi: 1056/AIoa2400802
- 14. Abd-Alrazaq AA, Alajlani M, Ali N, Denecke K, Bewick BM, Househ Perceptions and Opinions of Patients About Mental Health Chatbots: Scoping Review. J Med Internet Res 2021;23(1):e17828, doi: 10.2196/17828
- 15. American Medical Association: 2024 AMA Prior Authorization Survey, htps://www.ama-assn.org/system/files/prior-authorization-survey.pdf
- 16. Shah SJ, Crowell T, Jeong Y, et al. Physician Perspectives on Ambient AI Scribes. JAMA Netw 2025;8(3):e251904. doi:10.1001/jamanetworkopen.2025.1904
- 17. American Psychiatric Association: The Basics of Augmented Intelligence: Some Factors Psychiatrists Need to Know Now (2023), https://www.psychiatry.org/News-room/APA-Blogs/The-Basics-of-Augmented-Intelligence
- 18. Kleine A-K, Kokje E, Hummelsberger P, Lermer E, Schaffernak I, Gaube AI-enabled clinical decision support tools for mental healthcare: A product review. Artificial Intelligence in Medicine. 2025; Volume 160, Article103052. https://doi.org/10.1016/j.artmed.2024.103052.
- 19. Kleine A-K, Kokje E, Hummelsberger P, Lermer E, Schaffernak I, Gaube AI-enabled clinical decision support tools for mental healthcare: A product review. Artificial Intelligence in Medicine. 2025; Volume 160, Article103052. https://doi.org/10.1016/j.artmed.2024.103052.
- 20. Iyortsuun NK, Kim S-H, Jhon M, Yang H-J, Pant S. A Review of Machine Learning and Deep Learning Approaches on Mental Health Diagnosis. Healthcare. 2023; 11(3):285. https://doi.org/10.3390/healthcare11030285
- 21. Meinke C, Lueken U, Walter H, Hilbert K. Predicting treatment outcome based on resting-state functional connectivity in internalizing mental disorders: A systematic review and meta-analysis. Neuroscience & Biobehavioral Reviews. 2024; Volume 160, Article 105640, https://doi.org/10.1016/j.neubiorev.2024.105640.
- 22. Vieira A, Liang X, Guiomar R, Mechelli Can we predict who will benefit from cognitive-behavioural therapy? A systematic review and meta-analysis of machine learning studies. Clinical Psychology Review. 2022; Volume 97, Article 102193,
https://doi.org/10.1016/j.cpr.2022.102193.
- 23. Rony MKK, Das DC, Khatun MostT, et al. Artificial intelligence in psychiatry: A systematic review and meta-analysis of diagnostic and therapeutic efficacy. DIGITAL HEALTH. 2025;11. doi:10.1177/20552076251330528
- 24. He Y, Yang L, Qian C, Li T, Su Z, Zhang Q, Hou X. Conversational Agent Interventions for Mental Health Problems: Systematic Review and Meta-analysis of Randomized Controlled Trials. J Med Internet Res. 2023;25:e43862, doi: 10.2196/43862
- 25. Davenport T, Kalakota The potential for artificial intelligence in healthcare. Future Healthc J. 2019 Jun;6(2):94-98. doi: 10.7861/futurehosp.6-2-94
- 26. American Psychiatric Association: The Basics of Augmented Intelligence: Some Factors Psychiatrists Need to Know Now (2023), https://www.psychiatry.org/News-room/APA-Blogs/The-Basics-of-Augmented-Intelligence
- 27. Goldberg CB, Adams L, Blumenthal D, Brennan PF, Brown N, Butte AJ, Cheatham M, deBronkart D, Dixon J, Drazen J, Evans BJ, Hoffman SM, Holmes C, Lee P, Manrai AK, Omenn GS, Perlin JB, Ramoni R, Sapiro G, Sarkar R, Sood H, Vayena E, Kohane IS, for the RAISE Consortium. To Do No Harm — and the Most Good — with AI in Health Care. NEJM AI. 2024;1(3) doi: 10.1056/AIp2400036
- Adams L, Fontaine E, Lin S, Crowell T, Chung VCH, Gonzalez AA. Artificial Intelligence in Health, Health Care, and Biomedical Science: An AI Code of Conduct Principles and Commitments Discussion Draft. NAM Perspect. 2024 Apr 8;2024:10.31478/202403a. doi: 10.31478/202403a.